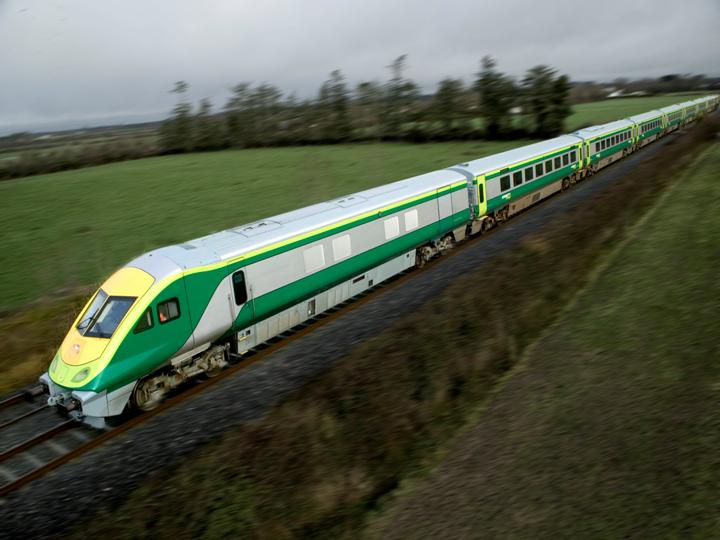
The value of predictive analytics in the railway sector
Olatz Iriondo, Business Development & Engineering, Ikusi
Technology provides powerful tools for the railway sector that are capable of capturing tens of thousands of data in real time, which can then be analysed to carry out, in a first stage, a descriptive analysis.
However, analysing this data can provide us with much more than that. At a higher-level stage, models can be created to gain in-depth knowledge of the behaviour of trains using predictive analytics which, based on different artificial intelligence techniques, makes it possible to describe reality and even to anticipate it.
Predictive analytics, which provides the ability to anticipate a breakdown in time to prevent it from happening, is a significant advancement on Condition-Based Maintenance (CBM).
A multitude of benefits can be obtained by incorporating this digital perspective into train operation. These benefits include minimised unplanned stoppages due to breakdowns, improved planning of maintenance and repair stoppages, reduced repair and inspection time, and continuous improvement in the life cycle of the train.
Beyond the analysis itself, and with the aim of generating the desired benefits, the first step is to determine the processes or areas in which predictive analytics can be useful. In the specific case of trains, we have identified three main areas: maintenance, operation and service.
The main contribution of predictive analytics in railway maintenance is efficiency, both in terms of the maintenance of the trains themselves and their on-board systems. Predictive analytics using artificial intelligence techniques helps with planning train and on-board systems maintenance, optimising the number of train service stops for maintenance. It also improves timetable planning and the maintenance tasks themselves.
Additionally, predictive analytics helps with train fleet operation, since it guarantees train managers the operational availability of the train through the early detection of possible anomalies in the train in operation, which the manager can then resolve, thus reducing the number of unplanned stops.
And, finally, it helps to carry out planning adapted to the needs of the service, by optimising operating costs.
In short, this is a valuable tool for the specific business needs of the railway sector which, like other business, seeks to provide the best service to users, in this case passengers. Predictive analytics contributes to this by optimising train maintenance and operation costs and processes through data analysis using artificial intelligence techniques.
For more information, please contact us: espana@www.ikusi.com